More than ever, small businesses are under increasing pressure to anticipate customer needs, optimise operations, and drive growth—all while working with limited resources.
The challenge of staying competitive can feel overwhelming, especially when larger companies leverage advanced analytics to make data-driven decisions.
Predictive analysis bridges this gap for small business owners, transforming raw data into valuable insights for smarter decision-making.
Yet, without the proper foundation—enough data, quality, and the correct tools—predictive analysis can seem like a complex, costly endeavour with little payoff.
A recent Salesforce survey found that 67% of small businesses using predictive analytics reported improvements in customer engagement and retention, underscoring its potential to drive meaningful results.
By investing in predictive analysis’s basics, even resource-limited businesses can improve their marketing, streamline inventory, and anticipate shifts in demand.
This guide will explore what it takes to make predictive analysis worthwhile for a small business.
From building a solid data foundation to choosing cost-effective tools, we’ll walk you through each critical component step-by-step.
Whether you are new to data analytics or looking to enhance your current approach, this post provides actionable insights to help you maximise predictive analysis without stretching your budget.
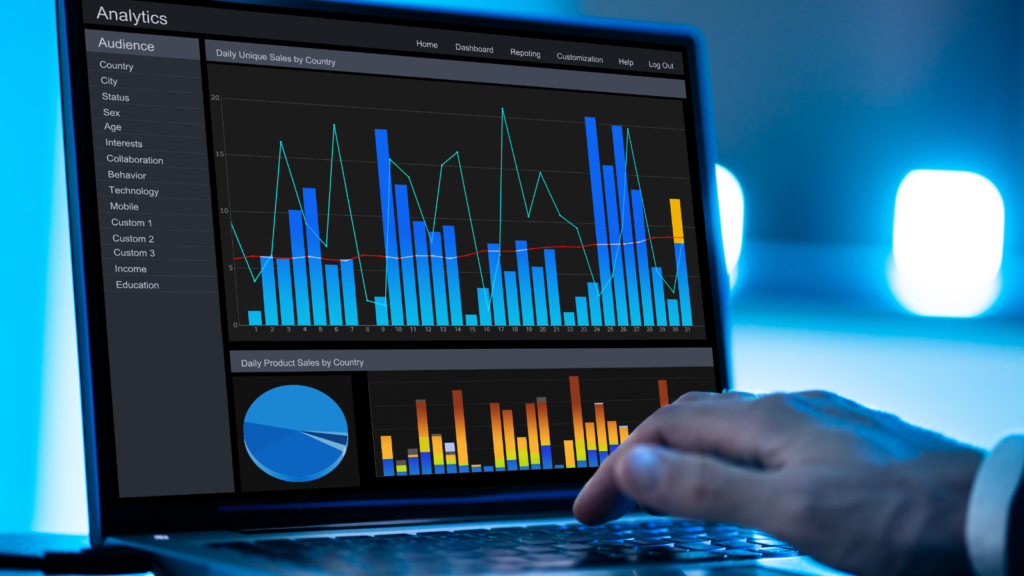
#1 What is Predictive Analysis, and Why Does It Matter for Small Businesses?
Predictive analysis uses historical data, statistical algorithms, and machine learning techniques to predict future outcomes.
For small businesses, this means leveraging data insights to make more informed decisions in marketing, inventory management, and customer retention.
Imagine being able to anticipate customer buying patterns, optimise stock levels for seasonal demands, or tailor marketing campaigns based on predicted trends—predictive analysis makes these scenarios possible, even on a small budget.
For many small business owners, the concept of predictive analysis might sound like something only large corporations can afford to do.
However, with the rise of user-friendly tools and more accessible data collection methods, predictive analysis is no longer exclusive to big players. Small businesses can now achieve significant results by making data-driven decisions, allowing them to compete more effectively in a crowded market.
Small businesses can focus on practical applications that drive real value without needing extensive data science resources by starting with a foundational understanding of predictive analysis.
The key advantage of predictive analysis lies in its potential to convert raw data into actionable insights. Instead of relying on guesswork, small businesses can base their strategies on likely future outcomes.
For example, a small retailer might analyse customer purchasing patterns over the last two years to forecast the upcoming season’s demand. This allows the business to adjust inventory levels accordingly, reducing the risk of stockouts or surplus inventory.
Moreover, predictive analysis supports more personalised customer experiences. By analysing data on customer behaviour, such as past purchases or browsing activity, a business can predict which products a customer is likely to buy next.
This insight enables small businesses to create targeted marketing campaigns that resonate with customers personally, ultimately boosting engagement and loyalty.
Implementing predictive analysis isn’t without its challenges. It requires a solid data foundation, reliable sources of information, and, most importantly, enough data points to ensure accuracy.
However, as we’ll explore in this guide, small businesses can start small and scale up as they gather more data and grow their capabilities.
#2 Data Volume: Why Size Matters
For predictive analysis to deliver valuable insights, small businesses need a sufficient volume of data.
The effectiveness of predictive models relies heavily on having enough data points to identify patterns, trends, and potential outcomes accurately. Without a critical mass of data, the results can be skewed, leading to more speculative predictions than accurate.
Reaching this “minimum data threshold” may require strategic planning for small businesses, especially if they’re just beginning to collect customer and operational data.
A good rule of thumb is to aim for a few thousand data points—whether transactions, customer interactions, or website visits—to make reliable predictions.
For example, if a business is trying to predict customer purchasing behaviour, having just a handful of transactions will likely yield inconsistent results. However, trends and patterns become much clearer with data from a few thousand interactions, allowing for actionable insights.
Starting small and gradually building up data volume over time is essential for smaller businesses, especially if they’re looking to implement predictive analysis on a budget.
Businesses can build this data volume by tracking daily interactions and touchpoints. Each customer purchase, website visit, or social media engagement contributes to the growing data pool that can be used for future predictions.
A simple customer relationship management (CRM) system can be invaluable for organising this data, even if the business isn’t ready to dive into full predictive analysis immediately. CRMs streamline data collection and improve data consistency, which is vital for accurate analysis.
In addition, small businesses should look for ways to increase the frequency and quality of their data. Engaging customers through loyalty programs, encouraging repeat purchases, and actively tracking website or app usage can all help to build a more robust dataset.
As the data pool grows, small businesses can unlock the full potential of predictive analysis to optimise inventory, tailor marketing strategies, and improve customer retention.
Tip
Start by setting up a Customer Relationship Management (CRM) system from day one to track customer interactions and organise data systematically. Even if predictive analysis isn’t immediately feasible, a CRM will help build data over time.
#3 Data Variety: The Power of Multiple Data Sources
While data volume is essential for accurate predictive analysis, data variety is equally crucial.
Relying on a single data type can limit the depth and scope of predictions, potentially leading to narrow or misleading insights. Small businesses can gain a more comprehensive view of customer behaviour and preferences by integrating multiple data sources—such as sales transactions, website interactions, customer demographics, and support inquiries.
This layered approach enriches predictive models, allowing them to account for various factors influencing customer decisions.
Each data source offers unique insights. Sales data can highlight purchasing trends, while website behaviour data reveals the types of content or products that attract customer interest.
Demographic data, on the other hand, helps segment customers by age, location, and lifestyle, making predictions more tailored and actionable.
Support interactions can even indicate areas where customers might need additional help or where a product or service might be improved.
When combined, these data types offer a holistic perspective, helping small businesses develop data-driven strategies from multiple angles.
Managing data from diverse sources may seem challenging, but today’s technology makes it more accessible than ever. Tools like Google Analytics, customer relationship management (CRM) systems, and e-commerce platforms provide user-friendly dashboards for gathering and organising data from multiple sources.
Many of these tools integrate seamlessly, allowing businesses to combine data without requiring complex technical skills or high-level data expertise.
Integrating data sources also contributes to greater accuracy in predictions. For example, a small business that uses sales and website behaviour data to predict demand will likely see more reliable results than one relying on sales data alone.
By analysing both data types, the business can better understand what customers buy and why they make those purchases.
These insights enable more precise demand forecasting, targeted marketing, and product recommendations, leading to higher customer satisfaction and more efficient operations.
Tip
Use integrated tools like Google Analytics and a CRM to combine data from various sources, ensuring a unified dataset for better analysis. Platforms supporting integrations make gathering and merging different data types easier.
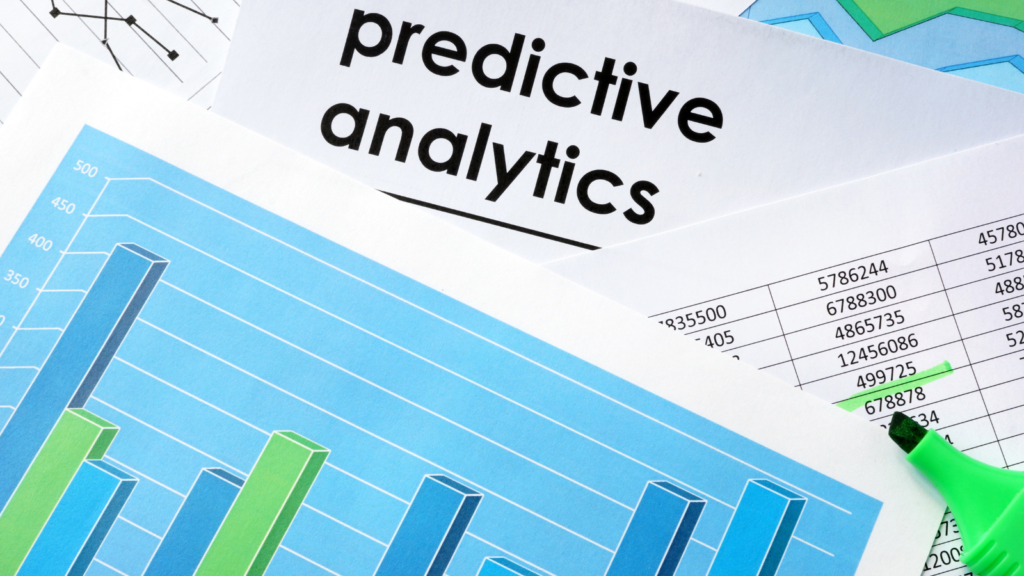
#4 Data Quality: Why Clean, Consistent Data is Essential
When it comes to predictive analysis, data quality is just as important as quantity. Even with thousands of data points, inaccurate or inconsistent data can lead to flawed predictions, ultimately wasting time and resources.
Maintaining high data quality is essential for small businesses aiming to make data-driven decisions. Clean, consistent data helps ensure that predictions are reliable and that the insights generated can genuinely inform business strategies.
Poor data quality often arises from inconsistencies, errors, or incomplete records. For example, if a business collects customer data without standardising entries—such as having multiple spellings of the same location or variations in format—it can result in duplicate records or skewed analytics.
Additionally, missing data, such as incomplete purchase histories or partial customer profiles, limits the ability of predictive models to produce accurate forecasts.
Small businesses can avoid these issues by focusing on data quality from the start and building a stronger foundation for analysis.
Regular data audits and standardised entry processes are two effective ways to maintain data quality. Conducting periodic checks for errors, duplicates, and inconsistencies ensures that the data remains accurate and actionable.
Implementing standardised data entry protocols—such as drop-down menus for categorical data or requiring specific formats for dates and phone numbers—can further reduce errors at the source.
For small businesses, these practices are manageable and yield significant benefits in the long run.
Another valuable tool in maintaining data quality is automation. Automated data-cleaning tools, like OpenRefine or built-in CRM functions, can help small businesses regularly clean and organise their data with minimal manual effort.
Automation reduces the risk of human error and ensures that data remains up-to-date, which is crucial for maintaining consistent predictive analysis accuracy.
Tip
Perform regular data audits and implement standardised data entry practices. Additionally, automated data-cleaning tools like OpenRefine can maintain data accuracy with minimal manual intervention.
#5 Customer Base Size: Building for Predictive Reliability
A solid customer base is a cornerstone for reliable predictive analysis. For small businesses, having a sufficient number of customers enables more accurate modelling and forecasting, as a larger data pool provides a broader and more representative sample.
Predictive analysis relies on identifying trends and patterns, which become clearer and more statistically reliable with more data points from a larger customer base.
Predictions can be skewed without enough data, leading to inaccurate insights and less effective strategies.
A practical threshold is a few hundred to a few thousand customers. While gaining some insights with smaller numbers is possible, predictive models thrive on more extensive datasets.
For example, a small business analysing the purchasing patterns of 500 customers will derive more robust and reliable insights than one with only 50. A larger customer base also allows businesses to segment data more effectively, enabling predictions tailored to different customer groups and improving overall targeting precision.
Growing a customer base is key to building a foundation for predictive analysis. Customer engagement strategies, such as loyalty programs, follow-up communications, and personalised offers, encourage repeat purchases and long-term relationships, adding more data points with each interaction.
Additionally, small businesses can incentivise customers to create accounts or sign up for newsletters, increasing the number of data touchpoints and enriching the dataset over time.
Long-term customer relationships boost predictive accuracy and yield deeper insights into customer behaviour patterns, preferences, and purchasing cycles.
With consistent data from repeat customers, small businesses can make predictions that account for individual preferences and behavioural changes over time.
For example, an e-commerce store could track the seasonal preferences of loyal customers, optimising inventory and marketing campaigns around those trends to maximise engagement.
Tip
Engage customers through loyalty programs and follow-ups to encourage repeat business. Building a loyal customer base increases the volume of data points, which supports more reliable predictions.
#6 The Right Tools and Technology: Accessible Solutions for Small Businesses
Implementing predictive analysis may sound complex, but small businesses can streamline the process without needing advanced data science expertise with the right tools and technology.
Thanks to the rise of user-friendly software and affordable analytics platforms, small businesses can now access tools that simplify data collection, storage, and analysis.
Investing in the right tools helps automate data organisation, supports seamless integration from multiple sources, and makes predictive analysis feasible on a smaller budget.
A variety of entry-level tools can meet the needs of small businesses, starting with predictive analytics. For instance, Google Analytics is a free tool that tracks website traffic and user behaviour, providing valuable data for understanding customer preferences and conversion trends.
Customer Relationship Management (CRM) platforms like HubSpot or Zoho allow businesses to track customer interactions, organise demographic data, and analyse purchase histories—all crucial for creating data-driven insights.
Additionally, some accounting and POS (Point of Sale) systems, like QuickBooks or Square, have built-in analytics features that help small businesses manage inventory and forecast sales based on past data.
Choosing tools that integrate well is key. Many of these platforms offer integrations with each other, allowing small businesses to combine data from multiple sources into a single, cohesive dataset.
This integration is vital for effective predictive analysis, as it ensures that data from sales, marketing, and customer service contribute to building a comprehensive view of customer behaviour.
For example, by connecting Google Analytics with a CRM platform, businesses can gain insights into how customers move from browsing to purchasing, enhancing the accuracy of their predictive models.
Budget-friendly and scalable tools are particularly advantageous for small businesses looking to start with basic predictive analysis and scale up as they grow.
Many CRM and analytics platforms offer tiered pricing, so businesses can begin with free or low-cost versions and upgrade as their data volume and analytical needs expand.
This gradual approach allows small businesses to stay within budget while building the foundation for more advanced predictive capabilities in the future.
Tip
Start with free or entry-level versions of CRM and analytics tools that offer scalability, like Google Analytics and HubSpot. Begin with basic tracking and upgrade as data volume and analytical needs grow.
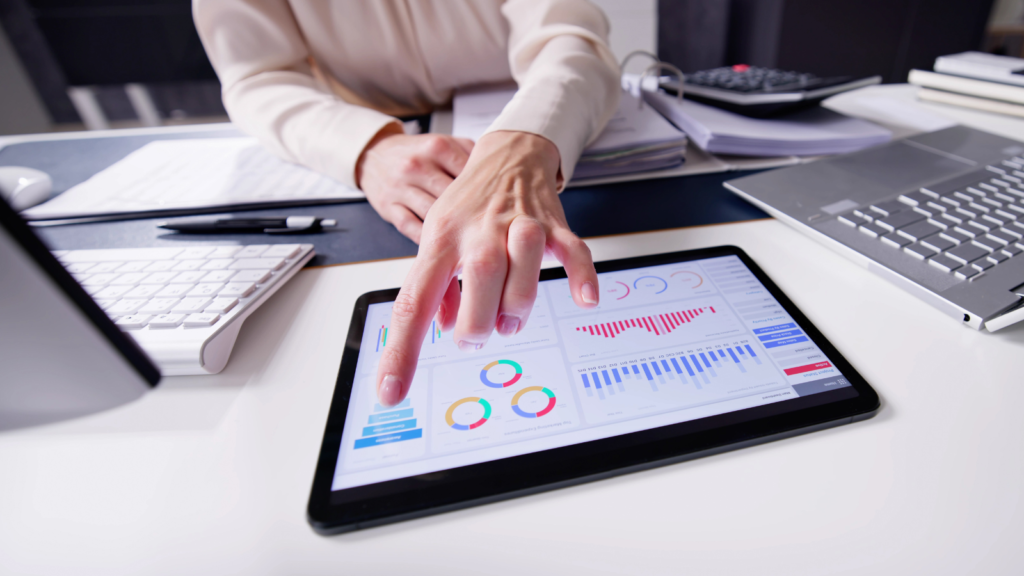
#7 When to Start Investing in Predictive Analytics
For small businesses, the decision to invest in predictive analytics should be timed to match data readiness, resource availability, and business growth objectives.
Predictive analysis doesn’t require a massive dataset or an advanced budget to get started, but it does need a solid foundation of reliable data and the right tools. Knowing the signs that your business is ready for predictive analytics can help maximise the return on this investment without overextending your resources.
One key indicator of readiness is data volume. If your business has consistently collected and stored customer, sales, and operational data, you’re already ahead. This baseline dataset enables you to start small with predictive analysis, using existing data to generate insights while gradually adding more as your business grows.
Another sign that it may be time to invest in predictive analytics is increased customer interactions or sales volume. More frequent interactions generate more data points, strengthening predictive accuracy and enabling businesses to forecast more precisely.
A consistent marketing budget and dedicated customer engagement efforts indicate that predictive analytics could add value. Businesses spending on regular marketing campaigns or loyalty programs can leverage predictive insights to optimise these investments.
For example, predictive analysis can help identify which customer segments are most likely to respond to a specific campaign or which promotions will drive the highest customer retention.
By focusing resources on data-backed strategies, small businesses can achieve better results and more efficiently use their budget.
Finally, it’s essential to set realistic expectations for predictive analytics. Starting small, with a limited set of predictions or basic forecasting, allows your business to test the waters without committing heavily upfront.
Over time, as you gain confidence and see results, you can scale up your analytics efforts, reinvesting in more advanced tools or complex predictive models.
Tip
Begin with basic predictions or limited forecasting to test the waters without heavy investment. Scale up analytics gradually based on your business’s growth and data accumulation, allowing a smoother transition into advanced predictive analysis.
Conclusion
Predictive analysis can be a game-changer for small businesses, transforming raw data into valuable insights that drive smarter, more strategic decisions.
From establishing a solid foundation with adequate data volume and variety to ensuring data quality and using the right tools, each step in the predictive analysis process brings small businesses closer to actionable, data-driven results.
A robust customer base and consistent historical data strengthen these predictions, enabling businesses to anticipate trends and optimise operations efficiently.
Small businesses can unlock predictive analysis’s potential without needing extensive resources by understanding the essentials—data volume, data variety, quality control, customer engagement, and scalable tools.
These foundational practices provide a practical approach to starting small and scaling up as data grows and capabilities expand.
Investing in predictive analysis at the right time can empower small businesses to stay competitive, improve customer retention, and make proactive decisions that support long-term growth.
Ready to leverage predictive analysis’s power for your business? Begin by setting up consistent data tracking and exploring affordable analytics tools that align with your goals.
Focus on building a reliable dataset and integrating multiple data sources, and you’ll soon see the benefits of data-driven decisions.
Dive into predictive analysis step-by-step and transform your data into a powerful asset for your business’s future success.
FAQs
Q1: What is predictive analysis, and how can it benefit my small business?
A1: Predictive analysis uses data, statistics, and machine learning to forecast future outcomes, helping small businesses make data-driven decisions. Benefits include more targeted marketing, improved inventory management, and better customer retention through personalised experiences. These insights enable businesses to anticipate trends, optimise resources, and increase efficiency.
Q2: How much data do I need to start with predictive analysis?
A2: A minimum of a few thousand data points—whether transactions, customer interactions, or website visits—is typically needed for accurate predictions. Building this volume takes time, so it’s essential to begin collecting and storing data early on through a CRM or other data-tracking systems.
Q3: Why is data variety important in predictive analysis?
A3: Data variety—using multiple data sources like sales, website behaviour, and customer demographics—provides a more comprehensive view of customer behaviour. This diversity helps predictive models account for different factors, improving accuracy and making insights more actionable.
Q4: How can small businesses maintain high data quality?
A4: Data quality is maintained by regular audits, consistent data entry, and automated data-cleaning tools like OpenRefine or CRM functions. Clean, standardized data helps avoid errors and ensures that predictions are reliable and useful for strategic decisions.
Q5: What are some affordable tools to help small businesses start with predictive analysis?
A5: There are many budget-friendly tools that support data tracking and basic analytics, including Google Analytics, HubSpot CRM, and QuickBooks. These tools offer scalable solutions, allowing small businesses to start with entry-level features and expand as they grow.
Q6: How do I know if my business is ready to invest in predictive analytics?
A6: Your business is likely ready for predictive analytics if you have a consistent data set, growing customer interactions, and a stable marketing budget. Start small by testing basic forecasting models and scale up as data grows and insights prove beneficial.
Q7: Can predictive analysis really make a difference for small businesses?
A7: Yes, studies show that data-driven businesses are more likely to achieve their goals. Predictive analysis helps small businesses stay competitive by enabling proactive, informed decisions, often leading to improved customer engagement, efficiency, and revenue growth.
Other Articles
How AI-Driven Market Research Gives You a Competitive Edge