Understanding consumer behaviour has become more challenging and crucial than ever.
Traditional research methods, while reliable, often struggle to keep pace with the sheer volume and complexity of data generated daily.
This is where AI-driven market research offers unprecedented insight into consumers’ intricate behaviours, preferences, and future actions.
By harnessing artificial intelligence, businesses can gain a sharper, deeper understanding of their customers. They can go beyond demographics to uncover the motivations and behaviours that drive purchasing decisions.
AI-driven consumer insights can be the remedy for those feeling frustrated by traditional research’s limitations. They offer the speed, precision, and scalability needed to stay ahead in today’s data-saturated world.
This post will explore how AI-powered tools and strategies transform market research, from predictive analytics forecasting trends to AI-driven customer segmentation, enabling personalised marketing like never before.
We’ll discuss the benefits, real-world applications, and best practices for implementing AI in market research so you can uncover deeper consumer insights and make informed, impactful business decisions.
Let’s dive into the future of market research and discover how AI can turn your data into a powerful advantage.
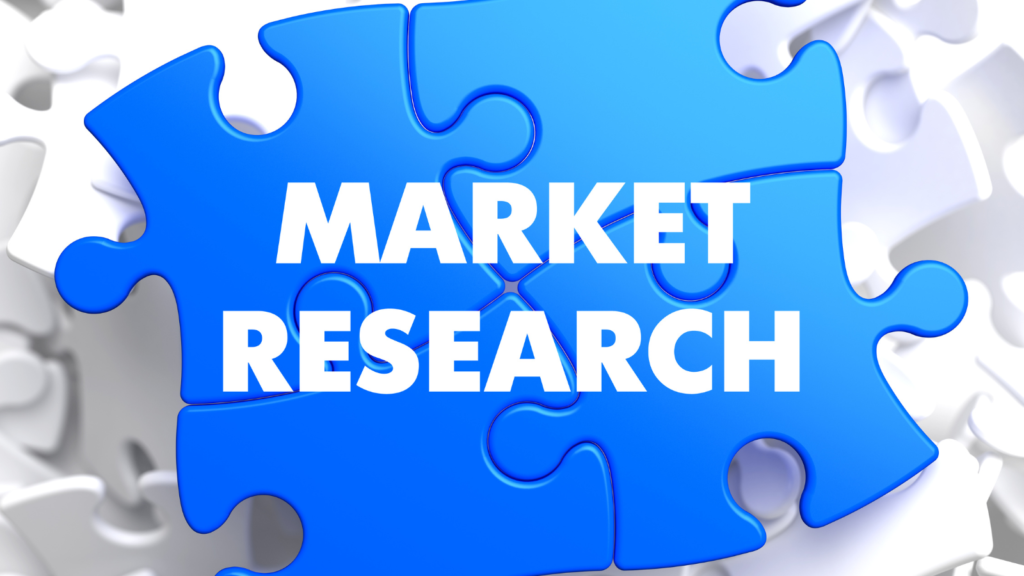
#1 Introduction to AI-Driven Market Research
As the volume of consumer data grows exponentially, traditional market research methods can often fall short.
Relying solely on surveys, focus groups, or historical data means missing today’s real-time, nuanced insights.
AI-driven market research provides a powerful alternative, leveraging machine learning, natural language processing, and predictive analytics to reveal consumer insights with unprecedented accuracy and speed.
AI doesn’t just analyse data; it interprets behaviour. Instead of merely capturing what consumers do, AI digs deeper, uncovering why they do it. This distinction allows businesses to move from broad demographic assumptions to more precisely understand consumer psychology, motivations, and preferences.
Imagine the difference between knowing that 30% of your customers are interested in eco-friendly products versus understanding which specific product features drive their choices—AI-driven research enables this level of insight.
How AI Transforms the Market Research Process
Speed and Scale
Traditional research methods are often time-consuming and limited by sample size. In contrast, AI can process massive datasets in real-time, analysing millions of data points from diverse sources like social media, online reviews, purchase history, and website interactions.
This capability allows businesses to get timely insights that adapt to changing market conditions.
Sentiment Analysis and NLP
With natural language processing (NLP), AI can analyse unstructured data—such as comments, reviews, or social media posts—transforming it into valuable sentiment insights.
By gauging consumer sentiment, AI helps businesses understand customers’ feelings about their brand, products, or competitors. This allows for proactive responses to feedback and shifting sentiments.
Predictive Power
AI doesn’t just look at past data; it predicts future behaviour. AI-driven predictive analytics can identify early indicators of emerging trends, helping brands stay ahead of consumer expectations.
For example, by identifying new trends in search behaviour or purchase patterns, brands can adjust their strategies to align with consumer interests before competitors catch on.
Personalised Consumer Insights
AI enables advanced segmentation beyond demographics, creating psychographic and behavioural segments. This personalised approach helps businesses understand customer preferences on a deeper level, allowing for marketing strategies that resonate with the motivations and values of specific groups.
#2 Why AI in Market Research? Key Benefits and Advantages
AI is revolutionising market research by unlocking insights that traditional methods alone cannot achieve.
AI-driven market research offers critical advantages, empowering businesses to gain a nuanced understanding of consumer behaviour, optimise marketing strategies, and stay ahead of the competition.
AI has become essential for companies looking to elevate their market research approach.
Deeper Behavioral Insights Beyond Demographics
While traditional market research can reveal demographic details—like age, gender, and income level—AI enables researchers to go beyond surface-level characteristics and uncover behavioural and psychographic insights.
AI systems analyse data from multiple sources, from social media interactions to online browsing patterns, to reveal motivations, preferences, and behaviours that aren’t visible through demographics alone.
This allows companies to understand the “why” behind consumer choices, creating more effective marketing strategies tailored to actual needs and desires.
Real-Time Data Processing for Timely Insights
Consumer behaviour evolves quickly, and responding to these shifts is vital for maintaining relevance. Unlike traditional research, which often involves waiting weeks for survey results and analysis, AI can process data in real-time.
This speed allows businesses to instantly identify and respond to emerging trends or changing consumer sentiments.
With AI, brands can adjust their marketing or product strategies on the fly, staying agile and responsive in dynamic markets.
Improved Accuracy Through Advanced Analytics
AI algorithms are designed to handle vast amounts of data with precision, reducing human error and eliminating biases inherent in manual data analysis. Machine learning models continually improve accuracy, allowing businesses to rely on data-driven conclusions.
This accuracy is particularly valuable in identifying subtle trends, such as shifts in brand sentiment or emerging product preferences that might otherwise go unnoticed.
Enhanced Personalization and Customer Targeting
AI-powered segmentation provides high personalisation, segmenting audiences based on complex behavioural patterns and preferences.
This enables brands to create highly targeted campaigns that speak directly to different audience segments.
For instance, a company could use AI to identify eco-conscious customers and target them with sustainable product offerings and messaging that align with their values.
This level of personalisation fosters stronger customer relationships and improves engagement and conversion rates.
#3 Top AI Market Research Tools and How They Drive Consumer Insights
AI-driven market research tools have transformed how companies understand and engage with their customers.
These tools leverage advanced technologies like machine learning, natural language processing (NLP), and predictive analytics to analyse vast amounts of data, providing actionable insights into consumer behaviour.
Here’s a look at some of the top AI-powered market research tools and how they drive consumer insights.
IBM Watson – Advanced NLP and Sentiment Analysis
IBM Watson is a leading AI tool known for its powerful NLP capabilities. It analyzes unstructured data such as social media posts, customer reviews, and survey responses to gauge consumer sentiment.
By understanding the emotions and attitudes behind customer feedback, businesses can identify areas for improvement and align their strategies with customer expectations.
Watson’s ability to analyse language at scale gives brands a clear picture of how they are perceived, helping them stay attuned to shifts in consumer sentiment.
Google Analytics 360 – Customer Journey Analysis
Google Analytics 360 goes beyond basic website metrics, offering insights into the customer journey.
Its AI-powered features track consumer behaviour across different touchpoints, identifying patterns that reveal how customers interact with brands online.
This tool’s machine learning capabilities can predict potential customer actions, enabling businesses to optimise their marketing strategies for each stage of the buying process.
By understanding the journey from awareness to purchase, companies can tailor experiences that meet customers’ where they are.
Crayon – Competitive Analysis and Market Monitoring
Crayon uses AI to conduct comprehensive competitive analysis by tracking competitors’ online presence, website changes, and customer engagement activities.
By collecting and analysing competitor data, Crayon allows brands to spot emerging trends and understand their competitors’ strategies.
This level of competitive intelligence can help businesses adjust their tactics quickly, ensuring they stay relevant and competitive in the market.
Qualtrics XM – Consumer Experience Management
Qualtrics XM is an AI-powered platform that focuses on managing consumer experience. It collects data from surveys, social media, and transactional feedback and uses machine learning to identify trends and predict consumer needs.
By aggregating and analysing experience data, Qualtrics helps companies improve customer satisfaction and retention, ultimately driving loyalty.
Its AI-driven insights guide brands in creating memorable, impactful consumer experiences tailored to specific segments.
Example
Unilever, one of the world’s largest consumer goods companies, uses IBM Watson to analyse millions of data points, including social media posts, reviews, and sales data.
With Watson’s sentiment analysis, Unilever gains insights into consumer preferences across global markets, allowing it to make data-backed decisions that improve product positioning and customer engagement.
By leveraging these top AI tools, companies can gain a comprehensive understanding of consumer behaviour, competitive positioning, and brand perception. Ultimately, these insights can drive growth and stay ahead in a competitive landscape.
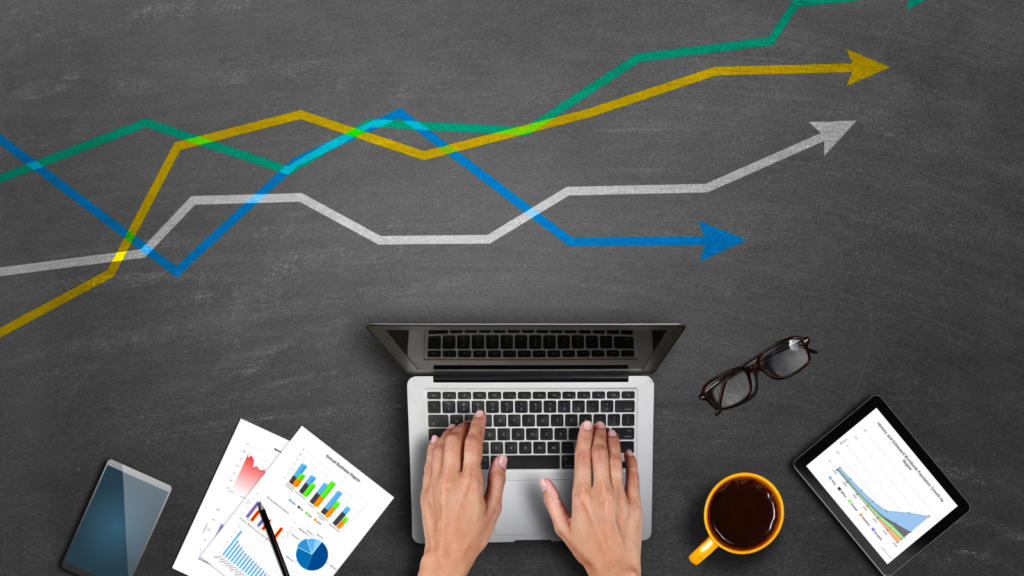
#4 Predictive Analytics and Consumer Behavior: Forecasting Trends with AI
In today’s competitive market, staying ahead of consumer trends is essential.
Predictive analytics powered by AI equips businesses to foresee shifts in consumer behaviour and proactively adjust their strategies.
By analysing historical and real-time data, AI-driven predictive models identify patterns that reveal future purchasing behaviours, enabling brands to respond to current demands and anticipate them.
Leveraging Search and Social Data for Early Trend Detection
AI-powered predictive analytics can analyse vast amounts of data from social media, search trends, and online interactions to identify emerging patterns.
By monitoring shifts in keywords, topics, and hashtags, AI systems can detect early signals of consumer interest or disinterest in particular products. This allows brands to adjust their messaging or introduce new products to meet emerging demand.
Identifying Purchase Intent Through Behavioral Data
Predictive analytics uses machine learning to examine behavioural data like browsing habits, past purchases, and engagement levels.
AI can accurately assess purchase intent by recognising patterns in consumer actions, such as frequently visiting certain product pages or engaging with specific content.
This insight helps brands time their promotions effectively, reaching consumers when they are most likely to convert.
Adapting Product Offerings with Demand Forecasting
AI-driven demand forecasting enables companies to anticipate future product demand based on historical data and current trends.
Predictive analytics informs inventory management and production schedules by evaluating seasonality, geographic preferences, and buying patterns.
This proactive approach minimises stock shortages or overstock situations, ensuring businesses are prepared to meet demand without incurring unnecessary costs.
Personalising Marketing Campaigns with Predictive Segmentation
Predictive analytics allows brands to segment customers based on predicted future behaviour rather than just past actions.
For instance, by identifying customers likely to switch to eco-friendly products, a brand can target these segments with messaging around sustainability and environmentally conscious options.
This level of personalised targeting improves engagement and strengthens brand loyalty by aligning with consumer values.
Example
Starbucks is a well-known example of predictive analytics in action. The company uses AI to track customer data, from purchase histories to location data, to predict what items customers are likely to order. Based on these predictions, Starbucks then tailors in-app offers and promotions, driving higher engagement and loyalty.
Starbucks has increased customer retention and satisfaction by personalising recommendations and rewards.
Through predictive analytics, brands can make informed, forward-thinking decisions that align with evolving consumer preferences, positioning themselves as market leaders in anticipating and meeting customer needs.
#5 AI-Powered Customer Segmentation: Personalizing the Consumer Experience
One of the most valuable applications of AI in market research is customer segmentation, which organises consumers into groups based on specific characteristics.
Traditional segmentation typically uses demographic data, such as age, location, or income, but AI goes much further.
Through advanced machine learning algorithms, AI can analyse behavioural, psychographic, and even real-time data to create more precise segments, allowing brands to deliver highly personalised experiences that resonate deeply with consumers.
Moving Beyond Demographics with Psychographic and Behavioral Data
With AI, brands can segment their audience based on psychographics, such as lifestyle, values, and interests, or behavioural data, like purchase history and website interactions.
This deeper segmentation reveals motivations that demographics alone can’t capture, enabling brands to target “who” their customers are and “why” they make certain choices.
For instance, a company might identify a segment of eco-conscious consumers who prioritise sustainable products and customise its messaging to appeal to their environmental values.
Real-Time Segmentation for Dynamic Consumer Targeting
AI-powered segmentation is dynamic, allowing businesses to adjust their segments in real-time based on new data.
By continuously analysing consumer actions, brands can update segments as behaviours shift, maintaining relevance in their messaging.
Real-time segmentation means that if a user starts browsing a new category of products, they can be immediately included in a relevant segment, enabling companies to deliver timely and targeted offers that resonate with the consumer’s current needs.
Personalised Marketing Strategies and Increased Engagement
Through more accurate segmentation, brands can develop hyper-targeted marketing strategies that speak directly to each group’s needs.
AI-powered segmentation has significantly improved customer engagement, as personalised messages are more likely to capture attention.
A skincare brand, for example, could identify a segment of customers with sensitive skin and offer them tailored product recommendations that align with their specific skin concerns, increasing engagement and conversion.
Driving Customer Loyalty Through Customized Experiences
AI-driven segmentation enhances acquisition and boosts loyalty. By continuously adapting to customer preferences and behaviours, brands can provide a unique and personal experience, strengthening customer relationships.
Personalised experiences make consumers feel understood, increasing the likelihood of staying loyal to the brand.
For instance, streaming platforms like Spotify use AI-driven segmentation to deliver personalised playlists, which fosters a more engaging and loyal user base.
Example
Spotify’s Discover Weekly playlist is a standout example of AI-driven segmentation at work. By analysing each user’s listening habits, Spotify creates personalised playlists based on individual tastes and preferences.
This segmentation strategy has proven highly effective, with over 40 million users regularly engaging with their Discover Weekly playlists. Spotify’s personalisation has become a defining feature of its service, driving user satisfaction and loyalty.
By leveraging AI-powered customer segmentation, brands can offer experiences that truly resonate, creating deeper connections with consumers and ultimately driving growth.
This precision and adaptability set AI-driven segmentation apart, helping brands build long-term loyalty in an increasingly competitive market.
#6 How Machine Learning Analyzes Buying Patterns and Preferences
Understanding what drives consumers to make purchasing decisions is at the core of effective market research.
Machine learning, a subset of AI, plays a transformative role here, uncovering insights beyond the reach of traditional analysis.
By examining unstructured data such as reviews, social media posts, and online behaviour, machine learning algorithms provide businesses with a nuanced view of consumer buying patterns and preferences.
Here’s how it works and why it’s so valuable.
Analysing Unstructured Data for Hidden Insights
Much consumer data—such as product reviews, social media comments, and customer service interactions—is unstructured, meaning it doesn’t fit neatly into rows and columns.
Machine learning, especially through natural language processing (NLP), can analyse this data to interpret customer sentiment, pain points, and preferences.
By understanding the language customers use when discussing products, businesses gain insights that allow them to address specific needs and improve customer satisfaction.
Identifying Buying Triggers and Influencing Factors
Machine learning helps brands identify the factors influencing purchasing decisions, such as product features, price sensitivity, or brand loyalty.
By analysing patterns in consumer behaviour, machine learning can uncover the common “triggers” that lead consumers to purchase.
For example, suppose a skincare brand discovers that customers tend to buy products after reading about ingredient benefits. In that case, they can emphasise this information in their marketing to capture attention and drive sales.
Personalising Recommendations with Predictive Models
Predictive modelling is a machine learning technique widely used to make personalised product recommendations.
E-commerce platforms like Amazon rely on machine learning to predict what customers might want to buy based on their past behaviour and preferences.
These recommendation systems analyse individual preferences and recommend items that align with each customer’s unique buying history, increasing the likelihood of conversion and enhancing the shopping experience.
Enabling Data-Driven Inventory and Product Planning
By tracking purchasing behaviour trends, machine learning also aids in inventory planning and product development.
For example, if machine learning models detect a rising interest in sustainable products, a brand can increase stock levels or introduce new eco-friendly options to meet demand.
This data-driven approach minimises the risk of overstocking or understocking products, optimises inventory, and ensures the availability of high-demand items.
Through machine learning, brands gain a deeper understanding of what influences purchasing decisions and can tailor their offerings and strategies accordingly.
This level of insight enables businesses to meet consumer expectations, optimise inventory, and deliver a more personalised shopping experience that builds loyalty and drives growth.
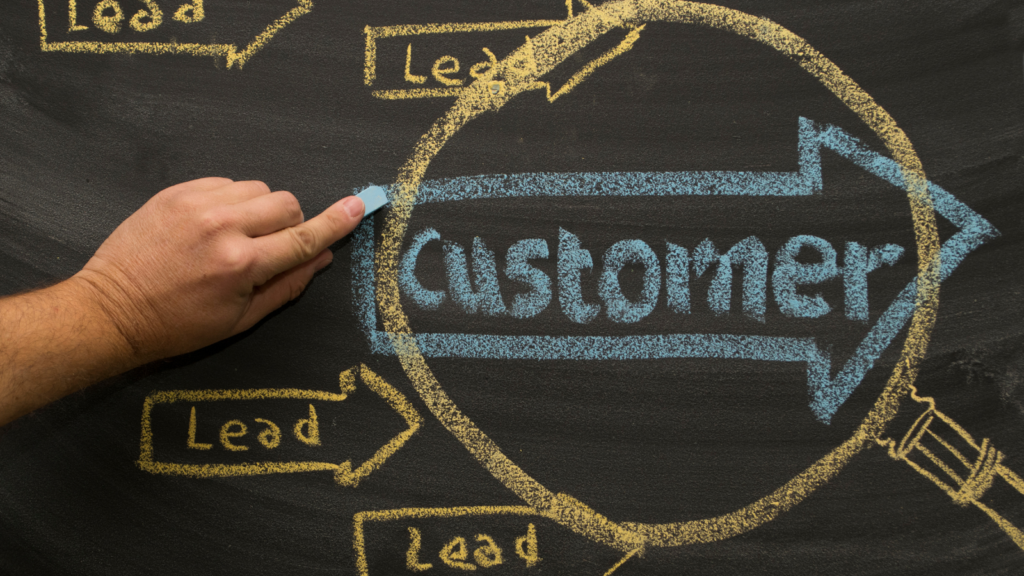
#7 Addressing Ethical Concerns in AI-Driven Consumer Analysis
While AI-driven market research offers valuable insights into consumer behaviour, it also brings ethical challenges.
Using AI to analyse consumer data raises important questions about privacy, transparency, and potential biases.
As brands leverage AI to better understand their customers, they must also consider how to use this technology responsibly, maintaining consumer trust and adhering to ethical standards.
Privacy and Data Protection Concerns
One of the primary ethical issues surrounding AI-driven consumer analysis is data privacy.
AI models often rely on vast amounts of consumer data, including personal details, purchasing history, and online behaviour. If mishandled, this data can lead to privacy breaches and harm consumer trust.
Brands must implement strict data protection protocols, ensuring that all collected data is used responsibly and in compliance with regulations like the General Data Protection Regulation (GDPR).
Ensuring Transparency and Consumer Consent
Transparency is crucial in maintaining ethical standards. Brands should be clear about collecting, storing, and analysing consumer data and allow users to consent to data usage.
This is particularly important as AI applications often work “behind the scenes,” and consumers may be unaware that their data is being analysed for insights.
Clear, accessible privacy policies and consent mechanisms help establish trust and ensure that consumers are informed participants in the data collection.
Mitigating Bias in AI Models
AI algorithms can unintentionally reinforce biases present in the data they are trained on.
For instance, if an AI model is trained on biased data, it may produce insights that disproportionately favour certain demographics, leading to unfair targeting or product recommendations.
Addressing this requires careful model design and testing, focusing on identifying and correcting biases.
Many brands now incorporate fairness checks into their AI systems to ensure equitable and inclusive analysis.
Ethical Use of Predictive Analytics and Behavioral Targeting
Predictive analytics allows brands to anticipate consumer needs and preferences, but there’s a fine line between helpful personalisation and invasive targeting.
Overly precise targeting can feel intrusive to consumers, leading to discomfort or suspicion about how much a brand “knows” about them.
Brands should strive to use predictive analytics to respect user boundaries, focusing on adding value to the consumer experience rather than merely maximising engagement or sales.
Example
The Cambridge Analytica scandal is a prominent case study of unethical data use, where AI-driven insights were derived from consumer data without proper consent.
This incident damaged consumer trust and led to increased regulatory scrutiny and calls for stricter data privacy laws.
Today, companies are more cautious, understanding the reputational and legal risks associated with unethical AI practices.
By addressing these ethical concerns, brands can ensure their use of AI in consumer analysis aligns with responsible data practices.
This approach protects consumers and helps build long-term trust, ensuring that AI-driven market research remains a valuable tool for understanding consumer needs in a way that respects their privacy and agency.
#8 Overcoming Challenges in Implementing AI in Market Research
Adopting AI-driven market research can bring transformative insights, but it’s not without its challenges.
Businesses often face hurdles from technical requirements to cost concerns when incorporating AI into their market research processes.
Understanding and learning how to overcome these challenges can make the difference between a successful AI implementation and one that falls short of expectations.
High Initial Costs and Resource Allocation
Implementing AI technology can be costly, requiring investments in both software and hardware.
In addition to purchasing or subscribing to AI tools, companies may need to upgrade their data infrastructure and invest in cloud computing resources to handle the data-intensive nature of AI applications.
Smaller companies, in particular, may find these upfront costs daunting.
To manage expenses, many businesses start with scalable, subscription-based AI solutions or partner with external AI vendors who can provide expertise and tools at a lower cost.
Data Quality and Integration Issues
AI’s effectiveness in market research depends heavily on data quality. Poor-quality data—such as outdated information, inaccuracies, or missing values—can lead to unreliable insights.
Moreover, integrating AI into existing systems can be complex, particularly if a company’s data is siloed across different platforms.
To overcome this, businesses should prioritise data cleaning and integration efforts before implementing AI, ensuring that the technology can access accurate, comprehensive data.
Using centralised data management systems can also streamline data access for AI analysis.
Lack of Skilled Talent and Expertise
The successful implementation of AI in market research requires specialised skills, including data science, machine learning, and knowledge of AI tools.
However, many companies struggle to find and retain employees with this expertise, particularly in smaller markets or industries where AI talent is scarce.
To bridge this gap, businesses can invest in training programs to upskill current employees or partner with AI consultants.
Alternatively, user-friendly, no-code AI platforms can help non-technical teams perform basic AI-driven research without extensive programming knowledge.
Adapting Organizational Culture and Processes
AI-driven market research often involves new processes and a shift towards data-driven decision-making, which may be unfamiliar or intimidating to some employees.
Resistance to change can be a significant barrier to AI adoption, as teams may be wary of relying on algorithms over traditional methods.
To address this, leadership should foster a data-driven culture by educating teams on the benefits of AI and encouraging experimentation.
Demonstrating early successes through pilot projects can also help build confidence and buy-in across the organisation.
Example
A well-known example of overcoming AI implementation challenges is Sephora, which integrated AI-driven personalisation into its e-commerce platform.
Sephora built a recommendation engine that enhances the online shopping experience by addressing data integration and investing in AI training.
This AI adoption contributed significantly to Sephora’s digital transformation, setting a standard in the beauty industry for data-driven personalisation.
By proactively tackling these challenges, businesses can set themselves up for successful AI adoption in market research.
Addressing these barriers enables companies to unlock AI’s full potential and achieve meaningful, data-backed insights into consumer behaviour, whether it’s finding cost-effective solutions, improving data quality, or building a data-driven culture.
Conclusion
AI has fundamentally transformed how businesses approach market research, and its influence will only grow.
From uncovering deep consumer insights to personalising marketing strategies and predicting future trends, AI-driven market research offers a competitive advantage in today’s data-driven world.
However, as powerful as AI is, it’s essential to recognise that technology alone isn’t enough; the human element—interpretation, strategic decision-making, and ethical consideration—remains crucial.
In summary, AI is redefining market research, providing the depth, speed, and precision needed to understand consumer behaviour at a new level.
By combining AI’s analytical power with human judgment and ethical practices, businesses can unlock the full potential of AI-driven consumer insights, positioning themselves for long-term success in an increasingly data-focused marketplace.
As we move into the future, companies that embrace this balance will lead the way in innovation, customer understanding, and market adaptation.
FAQs
Q1: What is AI-driven market research, and how does it differ from traditional market research?
A1: AI-driven market research uses artificial intelligence technologies, such as machine learning and natural language processing, to analyse vast amounts of consumer data quickly and with high accuracy. Unlike traditional methods that rely on manual data analysis and smaller data samples, AI can process large datasets in real-time, uncovering deeper insights into consumer behaviour, preferences, and emerging trends.
Q2: How can small businesses use AI in market research?
A2: Small businesses can leverage AI-powered tools like Google Analytics, HubSpot, and MonkeyLearn to gain insights without significant upfront costs. These tools offer features for behaviour analysis, customer segmentation, and sentiment analysis, making it easier for small companies to understand their audience, personalise marketing efforts, and optimise inventory management.
Q3: What are the ethical concerns related to AI-driven consumer analysis?
A3: Key ethical concerns include data privacy, transparency, and potential biases in AI algorithms. Businesses must handle consumer data responsibly, obtain clear consent, and ensure that AI models are trained on fair and representative data to avoid biased insights. Transparent data practices help maintain consumer trust and ensure responsible AI usage.
Q4: How does predictive analytics help in understanding consumer behaviour?
A4: Predictive analytics uses AI to analyse historical and real-time data, allowing businesses to forecast future consumer actions. By identifying patterns and trends, companies can anticipate consumer needs, optimise inventory, and personalise marketing efforts, ultimately leading to better engagement and higher customer satisfaction.
Q5: Can AI-powered tools replace human researchers in market research?
A5: AI is a powerful tool that enhances market research, but it cannot fully replace human researchers. While AI can handle data analysis at scale and provide detailed insights, human expertise is essential for interpreting findings, making strategic decisions, and considering the broader context. A balanced approach, where AI supports human decision-making, is often the most effective.
Q6: What are some examples of companies successfully using AI for consumer insights?
A6: Many companies use AI for market research and consumer insights. For instance, Amazon’s recommendation engine personalises product suggestions, driving 35% of its sales. Starbucks uses predictive analytics to customise in-app offers, while H&M leverages AI to optimise inventory based on trend analysis and demand forecasting.
Q7: Is AI in market research only beneficial for large companies?
A7: No, AI-driven market research is becoming accessible to businesses of all sizes. Many AI tools offer scalable and affordable options that allow small businesses to benefit from consumer insights, predictive analytics, and customer segmentation. As AI technology advances, more solutions are being tailored to meet the needs and budgets of small and medium-sized businesses.
Other Articles
A Proven Guide to Troubleshooting Your Digital Advertising
How to Use a KPI Dashboard to Drive Better Decisions
Measuring Your Marketing Success: Evaluating Outcomes for Growth