Bad data can have significant business consequences for companies. Poor-quality data is often pegged as the source of operational confusion, inaccurate analytics and ill-conceived business strategies.
The economic damage that data quality problems can cause include added expenses when products are shipped to the wrong customer addresses, lost sales opportunities because of erroneous or incomplete customer records, and fines for improper financial or regulatory compliance reporting.
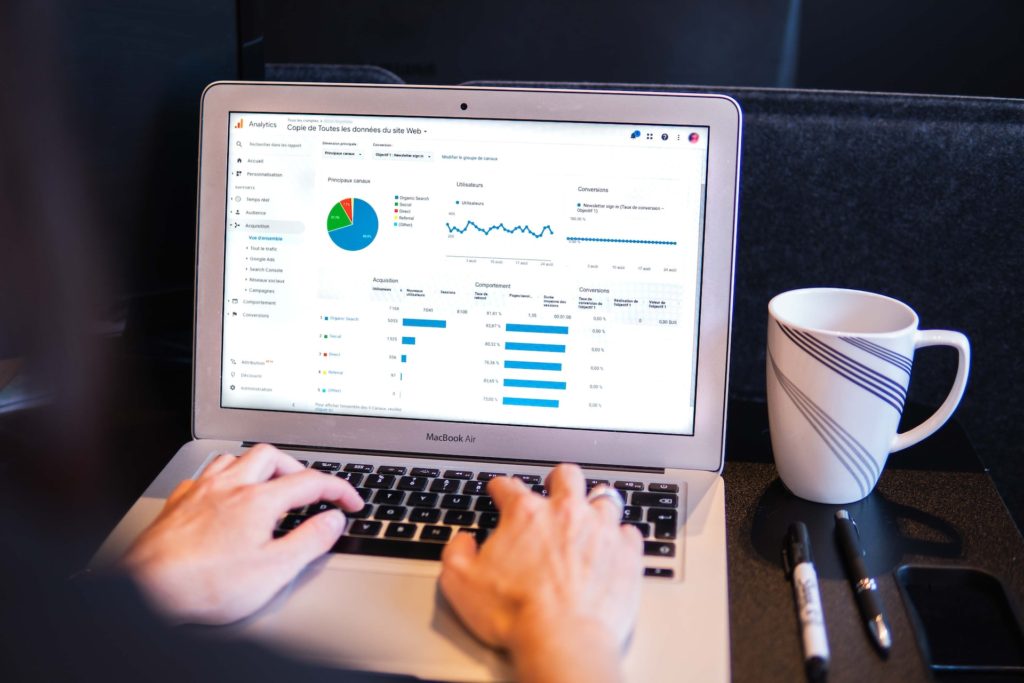
An oft-cited estimate by IBM calculated that the annual cost of data quality issues in the U.S. amounted to $3.1 trillion in 2016. In an article he wrote for the MIT Sloan Management Review in 2017, data quality consultant Thomas Redman estimated that correcting data errors and dealing with the business problems caused by bad data costs companies 15% to 25% of their annual revenue on average.
In addition, a lack of trust in data on the part of corporate executives and business managers is commonly cited among the chief impediments to using business intelligence ( BI ) and analytics tools to improve decision-making
Five Principles when Dealing with Data:
Accuracy:
This relates to the correctness of values contained in the various fields of the database record.
- Is the name spelled correctly?
- Are dollar amounts recorded properly?
Completeness:
Users must understand the data’s scope and be absolutely clear as to what comprises a particular data element – for example, “total revenue”.
Consistency:
Summarised information is in agreement with underlying detail.
Uniqueness:
One thing or entity in the real world must correspond to one and only one thing in your data. For example, XYZ Ltd and XYZ Limited represent the same entity in the real world, so one needs to be eliminated.
Timeliness:
Data must be current with respect to the needs of the business, and there should be systems in place for verified users to update or change data manually.
Good quality data is a crucial commodity that is not only desirable but necessary for managing projects, avoiding fraud, assessing performance, controlling finances and delivering services efficiently.
While data quality is important, it can often be pushed aside in the rush to manage all of your other responsibilities.
Give your business data the attention it needs, so you can benefit from better business decisions, better sales forecasts and better opportunities and deals.
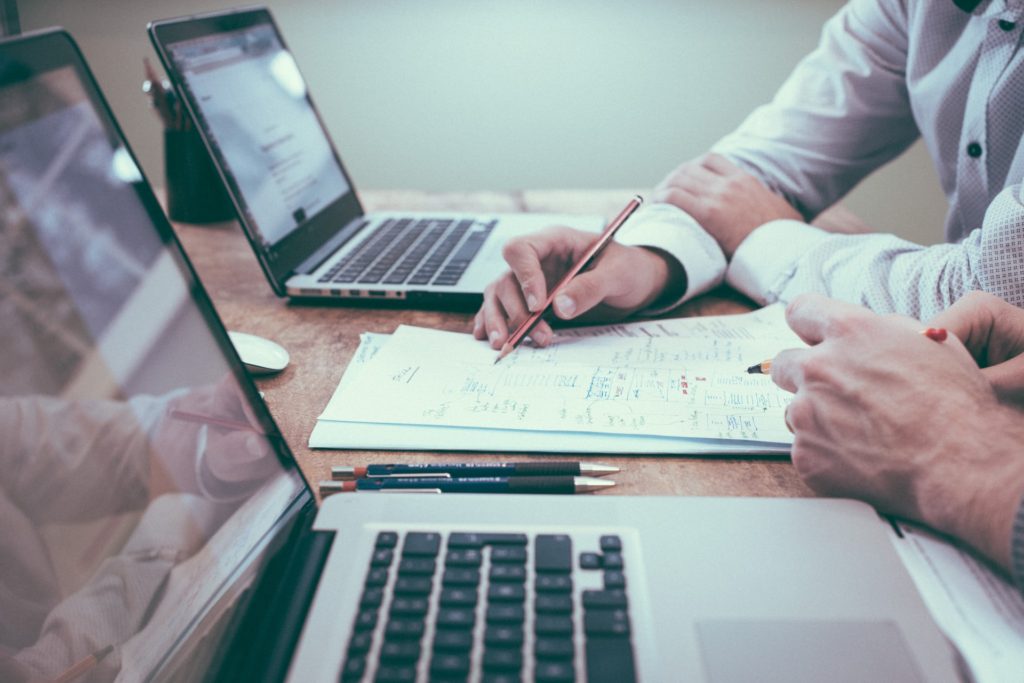
The implications poor data can have on your business are:
#1 Poor Decision-making
Poor-quality data leads to poor decisions. A decision can be no better than the information upon which it’s based, and critical decisions based on poor-quality data can have very serious consequences.
This is another reason why you should make sure that your data actually represents reality.
If your CRM contains insufficient prospect and client data, you’re making business decisions based on either best guesses or, worse, entirely incorrect information.
In today’s business climate, it is no longer acceptable to guide your business by gut instinct or intuition. Sure, those are still important, but a data-driven approach is imperative to ensure you’re providing the best guidance to your organization.
Business owners need good data in order to make good decisions (think GIGO). When marketing and sales teams have inaccurate information or outdated data in their databases, they are unable to make efficient decisions and ultimately waste resources.
For example, Forrester conducted research on how bad quality data affects marketing teams and their findings suggest that 21 cents of every media dollar spent were wasted due to poor data (about $16.5 million average annual loss for enterprises).
Ovum research estimates companies lose approximately 30% of revenue on average due to low data quality.
MIT and other research consultants suggest the cost of bad data can range from 15% to 25% of revenue.
#2 Business Inefficiencies
Poor-quality data causes inefficiencies in those business processes which depend on data from reports to ordering products and just about everything in between for which facts are required.
These inefficiencies may result in very expensive rework efforts validating and fixing data errors, instead of focusing on core duties.
The result of bad decisions is mistakes and more mistakes require more time to fix. It becomes a very tedious and painful process for the organization when people are unable to trust the data and have to spend resources to fix incorrect data.
All teams suffer productivity losses when an organization has poor-quality data. 32% of marketing teams’ time is spent on managing data quality and 26% of campaigns on average suffered from poor data quality.
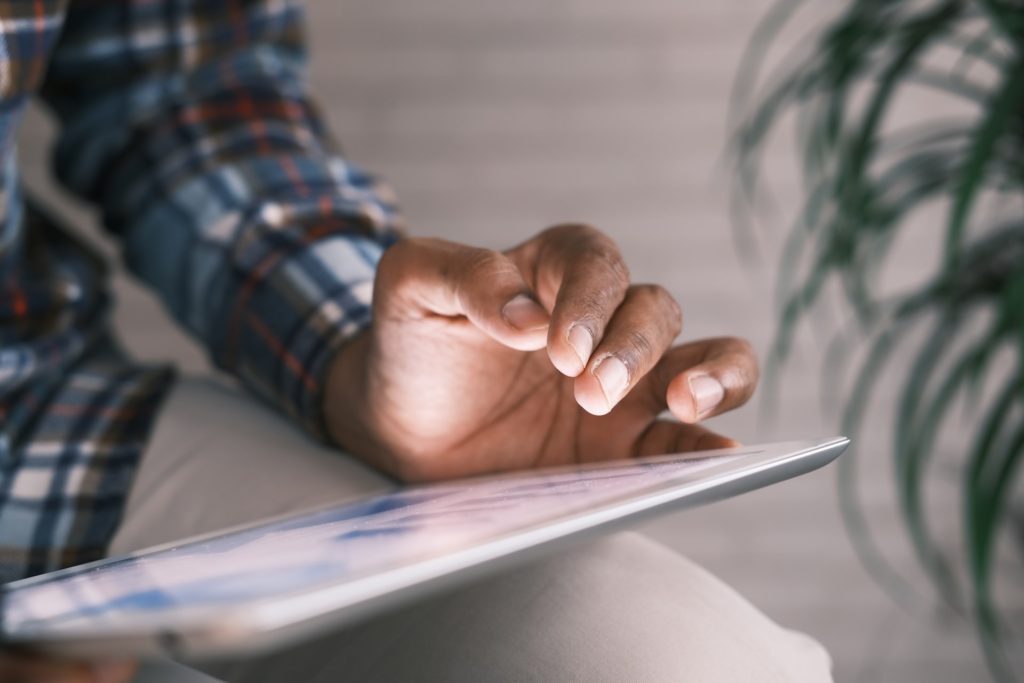
#3 Mistrust
Poor-quality data creates mistrust. Especially in industries where regulations govern relationships or trade with certain customers, such as finance.
Maintaining good-quality data can be the difference between compliance and millions of dollars in fines. If the data’s wrong, time, money, and reputations can be lost, reflecting adversely on your business and lowering customer confidence.
#4 Missed opportunities
A business may miss a lucrative opportunity for new product developments or customer needs that a competitor with a more advanced understanding of data may capitalise upon.
#5 Poor Service
Inaccurate CRM data has many ill effects, including the inability to properly segment contacts and companies, unclear sales forecasting, and poor hand-offs between marketing and sales.
#6 Inability to Identify Target Customers
An early step in building a marketing strategy is creating buyer personas or ideal customer profiles, semifictional representations of people or companies you’re going to target.
Combine empirical data from your CRM (e.g., job title, company size, tech stack) with anecdotal data from your team’s conversations with clients (such as pain points, common interaction types, and contacts’ preferred medium of communication).
If your CRM contains incomplete or inaccurate data, the conclusions you reach won’t be a true representation of your target market.
At the heart of inbound marketing is targeted and engaging content that’s created to draw qualified prospects to your site.
If you’ve made incorrect assumptions when creating target customer profiles, you’ll waste time and money creating messaging that doesn’t pertain to your target customers’ interests or needs.
In turn, your content won’t draw the traffic and engagement you’re looking for, or your team will waste resources by engaging with unqualified prospects.
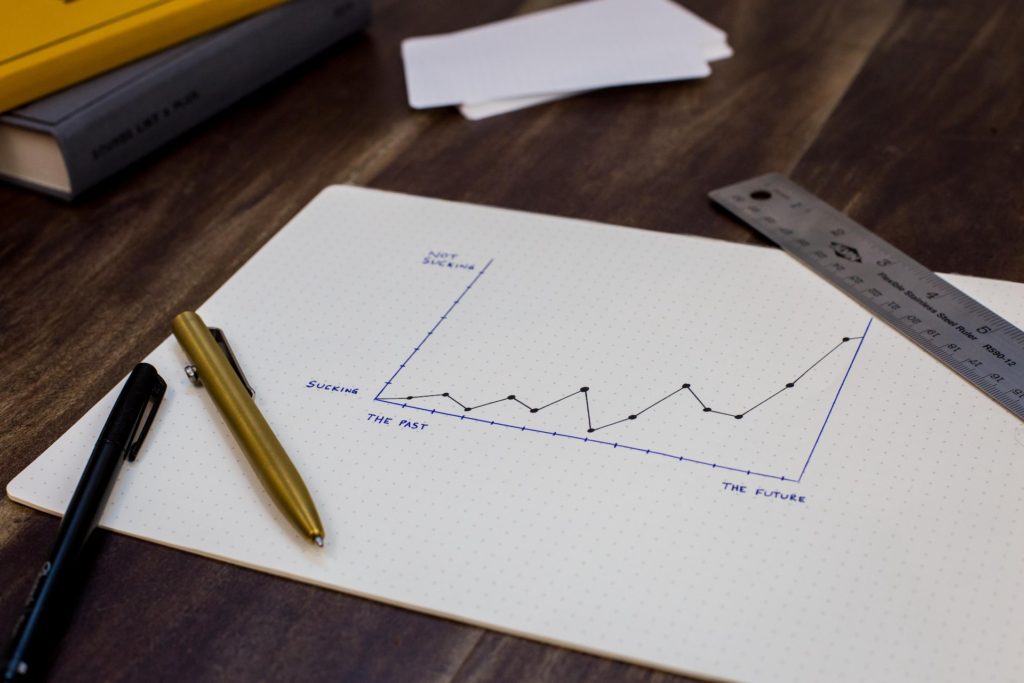
What does bad data actually cost businesses?
According to a Gartner survey, they found that 60% of businesses don’t know how much bad data actually costs them because they don’t measure the impact.
This indicates that a majority of businesses lack a fundamental understanding of how the quality of the data they have running through their systems affects their business outcomes.
What are the risks of poor data quality?
Simply put, you run the risk of losing money. Bad data and data decay weaken critical business activities, such as prospecting or running email campaigns.
Clean data enables your business to take focused action based on relevant data points. The more complete and accurate the data, the more likely it is that your marketing and sales efforts will align with the needs of your target customer.
What is the impact of bad data on sales and marketing?
The impact of dirty data on your sales and marketing teams can range from inaccurate targeting that prevents lead generation, to a sluggish sales pipeline that struggles to convert opportunities into customers.
Bad data also prevents successful automation. Many aspects of the sales and marketing process can be automated to optimize demand generation outreach. However, because automated email campaigns or sales call auto-dialling rely on data accuracy, they can misfire if based on bad data.
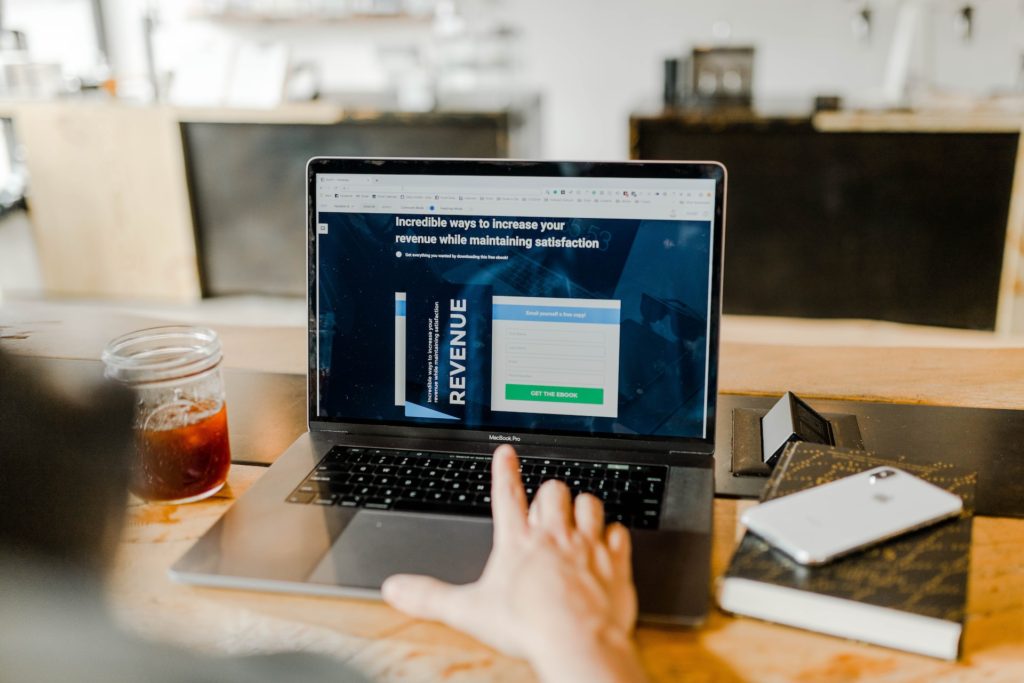
Four problems bad data causes for marketing activities
1. Possibility of getting blacklisted
Email marketing is the most ubiquitous form of marketing today. Ease of use, sophistication, and simplicity make it one of the best tools in a marketer’s arsenal.
However, email marketing with bad data on your email lists runs the risk of falling into spam traps. Spam traps are email addresses that have been set up by internet service providers to identify which senders are using lists with dirty data.
Too many trips into spam traps can get you blacklisted by your email service provider. In the worst case, they could suspend your email account. Your business then has to dedicate time and resources to solve this problem by either reversing the suspension or getting a new service provider.
2. Increased email churn
Email churn is caused by customer attrition. Churn rate refers to the percentage of email subscribers who leave your list over a period of time.
Churn refers to legitimate customers who were once on your list but have now either dropped your services and no longer wish to receive your organization’s emails or are no longer using the email address you used to contact them.
There are two types of list email churn:
Transparent churn: These are unsubscribes, hard bounces, and spam complaints.
Opaque churn: These are emails that land in a spam folder, go to an inactive email address, or remain unopened.
It’s important to factor churn into your database maintenance, otherwise, you run the risk of irritating people who don’t want to be on your marketing list. They may then take action and file spam complaints. In the long run, mismanaging churn can cause you to lose contact with new and existing customers and negatively impact your relationship-building efforts.
3. Prospects and customers get the wrong content
If your marketing database isn’t accurate, you might be sending prospects content that doesn’t align with where they are in the buyer’s journey.
For example, if the CEO of a company downloads your whitepaper, they may not appreciate getting an email notification to download the same document again. The marketing director of a prospective customer might grimace at emails addressed to “marketing specialist.” The same goes for names—Karla won’t be pleased to receive an email addressed to Carla.
Not only do these missteps directly affect your customers’ experiences, but they tarnish your brand reputation, too.
4. It wastes time
In B2B sales, and throughout your entire organisation, your salespeople are the most reliant on your CRM data.
According to recent studies, 94% of businesses suspect that the data they have on their customers is inaccurate. It’s been identified that chasing bad data wastes over 27.3% of a B2B salesperson’s time.
Malformed data is B2B data that is unusable or corrupt – like phone numbers with too many digits.
Malformed content in a contact field prevents salespeople from following up with leads. It also prevents searches from being populated with quality leads.
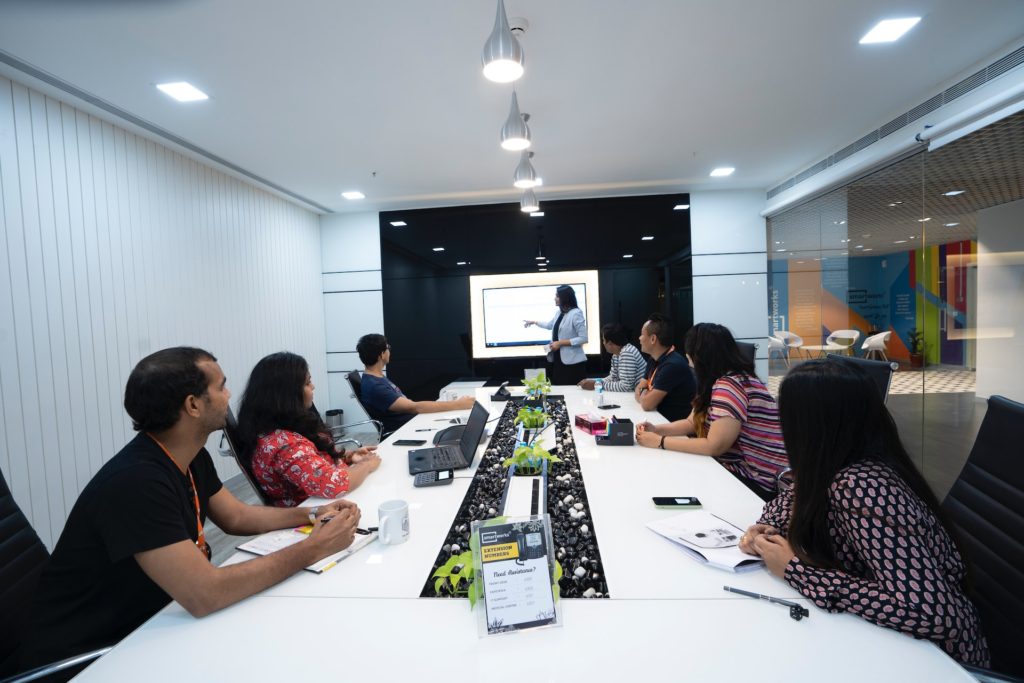
Two Other Serious Implications
Erroneous decisions made from bad data are not only inconvenient but also extremely costly. According to Gartner research, “the average financial impact of poor data quality on organizations is $9.7 million per year.” IBM also discovered that in the US alone, businesses lose $3.1 trillion annually due to poor data quality.
Productivity Cost
This all goes beyond dollars and cents.
Bad data slows employees down so much that they feel their performance suffers.
For example, every time a salesperson picks up the phone, they rely on the belief that they have the correct data – such as a phone number – of the person on the other end.
If they don’t, they’ve called a person that no longer exists at that number, something that wastes more than 27 per cent of their time.
Accommodating bad data is both time-consuming and expensive. The data needed may have plenty of errors, and in the face of a critical deadline, many individuals simply make corrections themselves to complete the task at hand.
Data quality is such a pervasive problem, in fact, that Forrester reports that nearly one-third of analysts spend more than 40 per cent of their time vetting and validating their analytics data before it can be used for strategic decision-making.
The crux of the problem is that as businesses grow, their business-critical data becomes fragmented.
There is no big picture because it’s scattered across applications, including on-premise applications. As all this change occurs, business-critical data becomes inconsistent, and no one knows which application has the most up-to-date information.
These issues sap productivity and force people to do too much manual work.
The New York Times noted that this can lead to what data scientists call ‘data wrangling’, ‘data munging’ and ‘data janitor’ work.
Data scientists, according to interviews and expert estimates, spend from 50 per cent to 80 per cent of their time mired in this more mundane labour of collecting and preparing unruly digital data, before it can be explored for useful nuggets.
Business Reputation
Poor data quality is not just a monetary problem; it can also damage a company’s reputation.
According to the Gartner report Measuring the Business Value of Data Quality, organizations make (often erroneous) assumptions about the state of their data and continue to experience inefficiencies, excessive costs, compliance risks and customer satisfaction issues as a result. In effect, data quality in their business goes unmanaged.
The impact on customer satisfaction undermines a company’s reputation, as customers can take to social media (as in the example at the opening of this article) to share their negative experiences. Employees too can start to question the validity of the underlying data when data inconsistencies are left unchecked. They may even ask a customer to validate product, service, and customer data during an interaction — increasing handle times and eroding trust.
Can you afford to continue with poor data in your business?